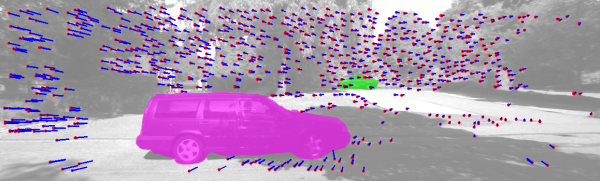
Role Of Vision Processing With Artificial Neural Networks In Autonomous Driving
In next 10 years, the automotive industry will bring more change than we have seen in the last 50, due to technological advancement. One of the largest changes will be the move to autonomous vehicles, usually known as the self-driving car. Scientists from many universities are striving to implement vision processing with the artificial neural network to provide driver assistance in self-driving cars.
Vision processing, as well as artificial neural networks, have been around for many years. Convolutional artificial neural networks (CNN) are sets of algorithms that extract meaningful information from sensor input. CNN’s are very computationally efficient at analyzing a scene. They are also able to identify objects as cars, people, animals, road signs, road junctions, road marking etc. enabling them to determine the relevant reality of the scene. As this system runs in real-time, the decision can be made as soon as the sensing part is complete.
One of the major steps in visual environment understanding for automotive applications is key points tracking and estimating ego-motion and environment structure subsequently from the trajectories of these key points. A propagation based tracking (PBT) method is popularly used to obtain the 2D trajectories from a sequence of images in a monocular camera setup.
The inputs from one or all of the sensors like LIDAR, RADAR, camera, IR, etc. are evaluated and decisions are taken accordingly. For example, if a car in the front suddenly brakes, the onboard computer would instantly verify the distance and calculate the speed with help of the existing sensors. Then it would apply the brakes faster than any human would be able to do. This method helps to prevent an accident with 90% efficiency.
The use of vision processing with CNN is rapidly increasing in automotive applications to enable camera-based autonomous driving. This technology sets a new driving standard. With this technology in our hand, fewer accidents, fewer fatalities, and less pollution are experienced. Vision processing in autonomous driving also enables efficient journeys, reduced crowding, car sharing, and packing cars in more tightly via vehicle to vehicle communication.